新利18体育新研究团队利用神经网络方法搜索强引力透镜系统
Searching strong lensing systems with the neural network technique
星系尺度的强引力透镜系统是重要的宇宙学探针,可用于深入地研究宇宙学和天体物理中的诸多科学问题,如暗物质性质、星系形成和演化以及哈勃常数的测量等。然而,目前已证认的强透镜系统数目过少,严重制约了相关天体物理学问题研究的开展。如何搜寻证认更多强透镜样本是当前工作中的主要问题。通过下一代大规模测光巡天项目的开展,人们期待发现数以万计的强透镜系统。但如何在海量的天体图像中快速地找到强透镜候选体?
近年来,人工智能的快速发展给我们提供了一种新的可能。国际上已有相关研究团队利用卷积神经网络方法搜索强引力透镜系统。在此基础上,新利18体育新的宇宙学团组构建并训练了一个卷积神经网络,并将其应用于欧洲南方天文台2.6米巡天望远镜(VST)千平方度巡天(Kilo-Degree Survey—KiDS)数据,发现了38个新的强透镜候选体。此外,通过测试卷积神经网络在不同观测条件上的表现以及用不同大小的训练集训练网络,该小组还对卷积神经网络的稳定性作了测试。该项工作构建的神经网络可应用于未来的其他巡天数据,如正在建设中的云南大学多通道测光巡天望远镜(Mephisto)的数据。
该项研究工作已被国际天文学主流期刊《英国皇家天文学会月刊》接受发表,论文第一作者是研究所硕士生何紫朝,指导老师为尔欣中教授和范祖辉教授(预印本链接:https://arxiv.org/abs/2007.00188)。
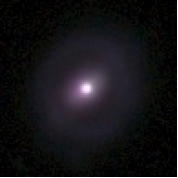
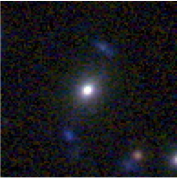
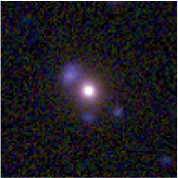
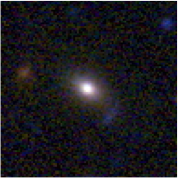
图1:新发现的38个强透镜候选体其中4个的图像。Figure 1: Images of 4 out of the 38 newly identified strong lensing candidates.

Galaxy-scale strong gravitational lensing systems serve as one of the most important probes to study a variety of problems in cosmology and astrophysics. For example, it allows us to probe the dark matter halos of galaxies, study the formation and evolution of galaxies, as well as to estimate the Hubble constant. However, the currently very limited samples of confirmed strong lenses have become the major bottleneck of such studies. With data from the next generation surveys, one expects to find tens of thousands strong lensing systems. Yet, how to identify robustly and efficiently strong lenses from the huge amount of imaging data?
The development of technique Convolutional Neural Network (CNN) has made rapid progress in recent years. The technique has been successfully applied to a variety of astronomical problems. The cosmology group at SWIFAR has constructed a CNN trained by simulated images of strong lensing systems. With this Network, they have identified 38 new strong lensing candidates in the KiDS (Kilo-Degree Survey) data collected with the European Southern Obervatory (ESO) 2.6 meter VLT Survey Telescope (VST). Moreover, the robustness of the CNN has been tested with respect to variations in the point spread function (PSF), training sample etc. The Network constructed in the current work has the potential to be applied to data of future surveys including that to be carried out by the Multi-channel Photometric Survey Telescope (Mephisto) currently being developed at SWIFAR.
The work has been accepted for publication by the MNRAS. The leading author of the paper, Mr. Zizhao He, is an M.Sc student of SWIFAR under the supervision of Profs. Xinzhong Er and Zuhui Fan. (https://arxiv.org/abs/2007.00188).